From humble beginnings of just ensuring uptime to a proactive approach in predicting and preventing IT issues, IT service management has come a long way. The evolution of ITSM practices has been further accelerated by cloud adoption, and now, the integration of AI and ML. A 2025 State of AI in IT report found that 40% of organizations that had adopted AI for ITSM reported improved employee productivity. Additionally, 33% reported enhanced user experience, 29% optimized operations and cost reduction, and 28% better decision-making.
Efficient ticket management fuels effective customer support and operations. Organizations field high volumes of service tickets that require swift categorization, prioritization, and resolution. And here is where Large Language Models, like GPT-4 and Gemini, help. LLMs, the backbone of Generative AI, are trained on a diverse and vast amount of data to process, understand and generate human-like language. This translates to automating and optimizing ticket management.
For instance, LLMs can automatically categorize incoming tickets based on their content, directing them to the appropriate teams or departments with unparalleled accuracy. They can also summarize lengthy ticket descriptions, enabling support agents to grasp the core issues at a glance and respond more efficiently. Beyond that, LLMs excel at generating coherent and context-aware responses, tackling repetitive queries while freeing up human agents to focus on more complex issues. In this blog, we take a look at the Tiger Analytics approach to using LLMs for smarter ticket handling and how it can help organizations enhance operational efficiency.
The Tiger Analytics Approach to LLMs for ITSM
At Tiger Analytics, we partnered with several clients to streamline the entire ticket management process using LLMs. Together, we built solutions that integrate with the client’s existing service management tools while providing an additional layer of intelligence. The crux of Tiger Analytics’ approach can be condensed into four pillars.
We found that leveraging LLMs led to tangible improvements in how service management teams handle tickets. Intelligent automation optimized the resolution process, and also empowered service teams to focus on more complex issues. We observed the following key benefits realized across clients:
- 15% to 20% of tickets resolved via automated self-service.
- ~95% accuracy of results across different modules.
- Significant reduction in time taken for resolution of critical and priority tickets.
How LLMs enhance key processes in the ticket lifecycle
Now, we take a closer look at how integrating LLMs into four processes of an IT helpdesk can prove beneficial.
1. Efficient Triaging: Routing tickets to the right teams
No more manual sorting – The GenAI system analyzes historical ticket data to automatically route incoming tickets to the appropriate support teams. This ensures faster response times and reduces the risk of misrouted tickets. Furthermore, the system can assign tickets to available agents within those teams, ensuring even workload distribution. The LLM’s reasoning for the chosen team and agent is also made visible to promote transparency.
2. Intelligent Prioritization: Focusing on what matters most
By analyzing ticket data, the module can intelligently prioritize incidents based on their urgency and potential business impact. Here’s how it works:
- Sentiment analysis: The LLM assesses the user’s emotional tone in the ticket description to gauge the issue’s severity.
- Business value identification: It determines the affected systems or services and their importance to the organization using the ticket metadata.
- Combined priority analysis: The LLM combines sentiment and business value data to assign an overall priority level.
- Transparent thought process: The LLM’s reasoning behind the assigned priority is made available, promoting understanding and trust in the system.
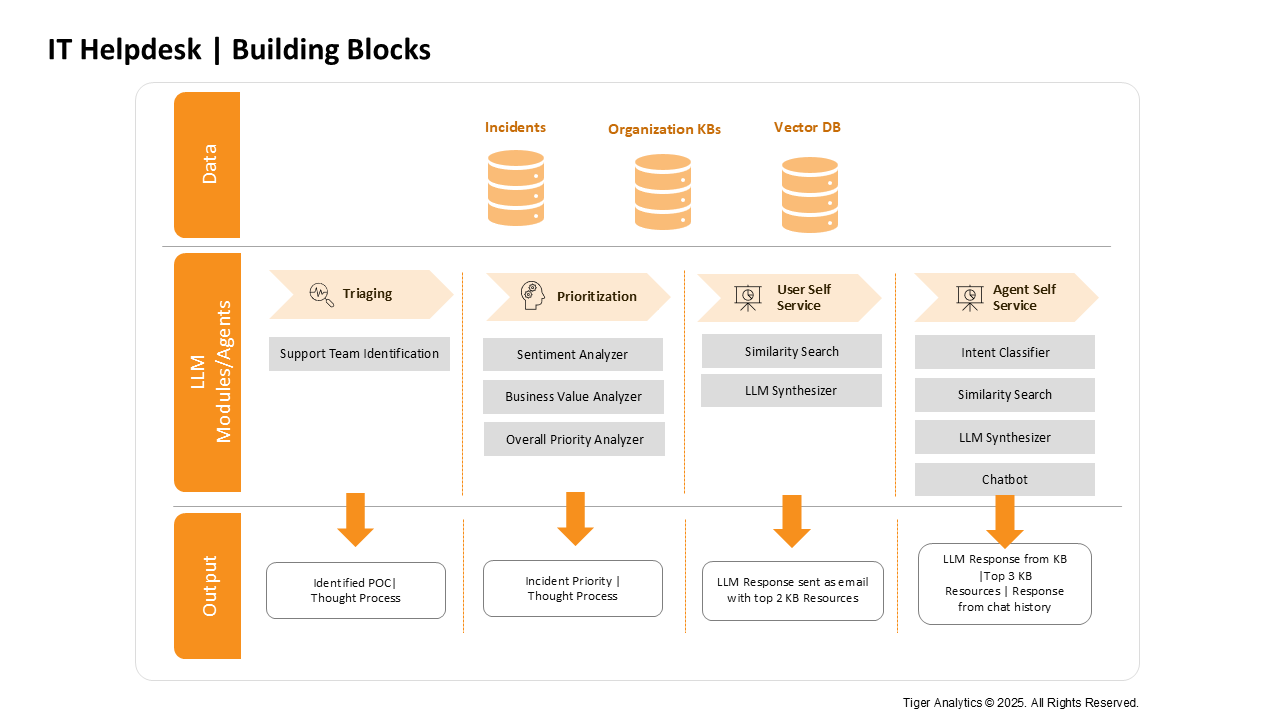
3. User Self-Service Incident Resolution: Empowering users and reducing agent workload
This module aims to empower users to resolve their issues independently, thus reducing the burden on IT agents. Consider a scenario where a user encounters a common issue like password reset. The LLM-powered system can:
- Identify the user’s intent from their initial ticket submission (e.g., email or form).
- Retrieve relevant information from a knowledge base using Retrieval Augmented Generation (RAG).
- Provide step-by-step instructions to the user via email or integrated communication channels.
- Track ticket status and send automated reminders for pending user actions.
- Allow users to provide feedback on the resolution process.
For more complex or high-priority tickets, the system automatically routes them to human agents by first assessing the priority of the ticket and then triaging for right assignment.
4. Agent Self-Service: Enabling agents with instant knowledge
This solution extends AI capabilities to assist agents in resolving tickets quickly and efficiently. An LLM-powered chatbot is integrated into the agent’s workflow, allowing them to:
- Query the knowledge base: Agents can use natural language to ask questions about specific issues or procedures.
- Retrieve relevant documentation: The chatbot uses similarity search to identify the most relevant articles or guides from the knowledge base.
- Obtain concise summaries: The LLM summarizes complex documentation into easily digestible answers for agents.
- Engage in multi-turn conversations: Agents can ask follow-up questions to clarify doubts or delve deeper into a topic.
- Access ticket metadata: The chatbot provides instant access to relevant ticket information, reducing the need for manual searches.
- Handle out-of-scope queries: The system is designed to efficiently handle questions that fall outside its knowledge domain.
Integrating LLMs into IT ticket management empowers both users and agents, streamlines workflows, and ensures faster, more efficient resolutions. These powerful tools also help enhance overall user experience and optimize resource allocation, freeing up valuable time for agents to focus on more complex and strategic tasks.